Clinical Endpoint Results & LLMs
Authors: Lydia Frick, Daniel Brand, Heike Kielhorn-Schönermark, Matthias Schönermark
Poster presentation at ISPOR Europe 2024, Barcelona, Spain
Value in Health, Volume 27, Issue 12, S2 (December 2024)
Objectives:
With the EU HTA process, new challenges arise for the HTA dossier compilation due to the expected huge number of PICO schemes to be addressed in only 100 days from notification about the PICO schemes and dossier submission. Mastering this process operatively requires new and tech-enabled approaches. There is a high potential for large language models (LLM) to support dossier compilation, such as for description and interpretation of endpoint results.
Methods
Ten quality criteria for the generated description were defined, such as “no hallucinations” and “all numbers are correct”. Additionally, five conventions regarding content and structure of the generated text were defined based on extensive experience in German HTA dossier compilation. For the development dataset, 1,264 tables from publicly available German AMNOG dossiers were catalogued and categorized resulting in 15 table types. For each table type, a set of synthetic tables was generated to feed into a core algorithm operating PaLM 2 32k text-bison allowing for basic table understanding, imitation of writing style and fine-grained control of the LLM output. 245 tables were transformed into machine-readable format used as input for the LLM algorithm. The LLM outputs were evaluated regarding the need for adjustment to identify and categorize mistakes.
Results
During the 5-week piloting phase, 47% of the generated results were directly usable or required minor adjustments, 35% of the generated descriptions required major adjustments but were still helpful, and 19% of the descriptions were not helpful. Most abundantly, data extraction from the table was incomplete or wording and writing style required adjustments. Importantly, hallucination was not identified as a major concern as shown by a low hallucination score for the extracted data.
Conclusions
Commodity LLMs can describe endpoint table results accurately, across a meaningful set of different tables and at a sufficient level of sophistication required for HTA and other purposes.
Kontaktieren Sie uns
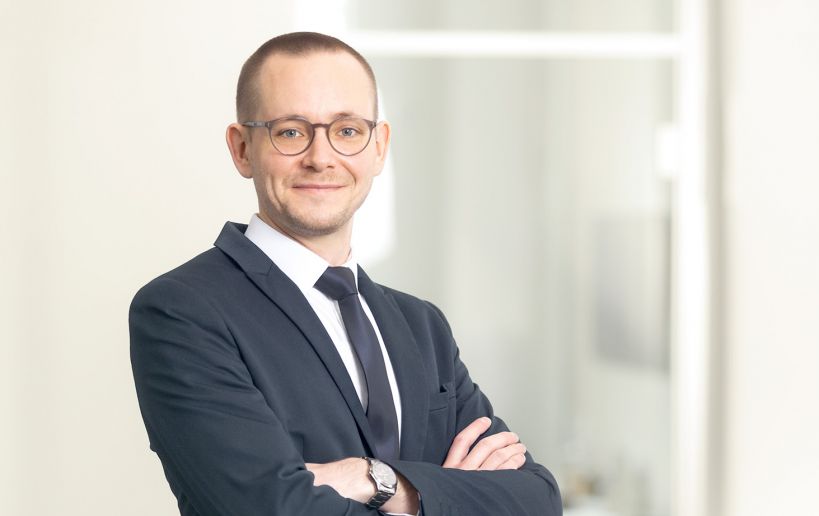
Senior Medical Writer
M.Sc. Biomedizin
Fax: +49 511 64 68 14 – 18
Hier stellen wir Ihnen unsere englisches Poster als kostenlosen Download zur Verfügung.